Building A Development Spark Cluster with Postgres Using Docker And Make
Overview
My goal is to build a decent approximation of a production Apache Spark EMR environment for development and testing.
For development, I need:
- A Postgres database to persist datasets.
- A Spark cluster with two worker nodes.
- A Jupiter notebook server for prototyping.
- To submit a PySpark job to the Spark master.
- A Shared filesystem for file processing and code sharing.
The approach I've taken is to run everything on Docker to avoid building up an environment from scratch.
The Docker setup has a develop
network where all of the containers run. To support development, I've exposed the Spark UI, Jupter and Postgres ports. Spark UI is exposed on 8100 and Jupyter is on 9999, I use non-standard ports to avoid conflicts with other stuff on the host machine.
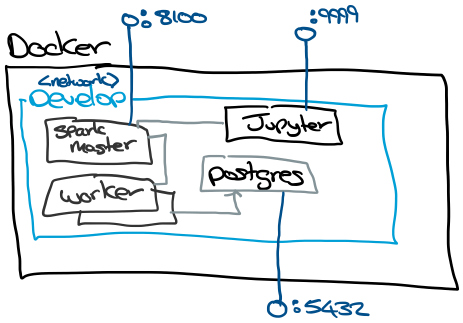
Building the environment
# Clone the repo
git clone https://github.com/omahoco/spark-postgres.git
# Create the environment
cd spark-postgres
make all
Testing via Jupyter
Navigate to http://localhost:9999. Jupyter will ask you for a token for authentication.
To get the authentication token from the Jupiter server.
cd spark-postgres
make jupyter_token
In Jupyter, you can test connectivity to the spark master using the following code.
import pyspark
conf = pyspark.SparkConf().setAppName('Jupyter').setMaster('spark://spark:7077')
sc = pyspark.SparkContext(conf=conf)
session = pyspark.sql.SparkSession(sc)
rdd = sc.emptyRDD()
print(rdd.isEmpty())
sc.stop()
Next, let's test connectivity to Postgres by counting the number of Postgres users.
import pyspark
conf = pyspark.SparkConf().setAppName('Postgres').setMaster('spark://spark:7077')
sc = pyspark.SparkContext(conf=conf)
session = pyspark.sql.SparkSession(sc)
jdbc_url = 'jdbc:postgresql://postgres/postgres'
connection_properties = {
'user': 'postgres',
'password': 'postgres',
'driver': 'org.postgresql.Driver',
'stringtype': 'unspecified'}
df = session.read.jdbc(jdbc_url,'pg_catalog.pg_user',properties=connection_properties)
print(df.count())
sc.stop()
Spark Working With Files
The Spark and Jupyter containers mount the host's /tmp
folder to /data
. The mount acts as a shared file system accessible to both Jupyter and Spark. In a production environment a network file system, S3 or HDFS would be used.
In the example below, we download the dataset and ask Spark to load it into a dataframe. Next, we use an aggerate function to calculate the average systolic blood pressure from the sample.
From a Jupyter Notebook:
!curl https://raw.githubusercontent.com/omahoco/spark-postgres/master/nhanes_2015_2016.csv -O /data/nhanes_2015_2016.csv
import pyspark
conf = pyspark.SparkConf().setAppName('Jupyter').setMaster('spark://spark:7077')
sc = pyspark.SparkContext(conf=conf)
session = pyspark.sql.SparkSession(sc)
# Load Some NHANYES Data - https://www.cdc.gov/nchs/nhanes/index.htm
nhanyes_df = session.read.format('csv').option("header", "true").load('/data/nhanes_2015_2016.csv')
from pyspark.sql.functions import mean as _mean, stddev as _stddev, col
# BPXSY2- Systolic: Blood pressure (second reading) mm Hg
stats_df = nhanyes_df.select(
_mean(col('BPXSY2')).alias('BPXSY2_mean'),
_stddev(col('BPXSY2')).alias('BPXSY2_std')
).collect()
stats_df
The output should be [Row(BPXSY2_mean=124.78301716350497, BPXSY2_std=18.527011720294993)]
Spark Working With Tables
In this example, we will create a Postgres table to track virtual coin tosses. We will use the table and Spark to calculate the frequency we get heads or tails over a million coin tosses.
Creating Random Data
Connect to Postgres and get a terminal to enter interactive queries
docker exec -it postgres /bin/bash
psql --user postgres
Inside psql
let's generate 1 million random coin toss events.
CREATE TABLE public.coin_toss (
event_ts timestamp default now(),
outcome TEXT NOT NULL
);
INSERT INTO public.coin_toss(outcome)
SELECT CASE WHEN RANDOM() < 0.5 THEN 'heads' ELSE 'tails' END FROM generate_series(1, 1000000);
Calculating the Frequencies With Spark
import pyspark
conf = pyspark.SparkConf().setAppName('Postgres').setMaster('spark://spark:7077')
sc = pyspark.SparkContext(conf=conf)
session = pyspark.sql.SparkSession(sc)
jdbc_url = 'jdbc:postgresql://postgres/postgres'
connection_properties = {
'user': 'postgres',
'password': 'postgres',
'driver': 'org.postgresql.Driver',
'stringtype': 'unspecified'}
df = session.read.jdbc(jdbc_url,'public.coin_toss',properties=connection_properties)
samples = df.count()
stats = df.groupBy('outcome').count()
for row in stats.rdd.collect():
print("{} {}%".format(row['outcome'], row['count'] / samples * 100))
sc.stop()
Running A Spark Job
Instead of driving everything from a Jupyter notebook, we can run our Python code directly on Spark by submitting it as a job.
I've packaged the coin toss example as a Python file at pyspark/src/main.py
.
We can submit the code as a job to Spark by running:
make spark-submit